Clustering in machine learning is an unsupervised learning set of algorithms that divide objects into similar clusters based on similar characteristics.
What is Clustering in Machine Learning?
Clustering is used to group similar data points together based on their characteristics.
Clustering machine-learning algorithms are grouping similar elements in such a way that the distance between each element of the cluster are closer to each other than to any other cluster.
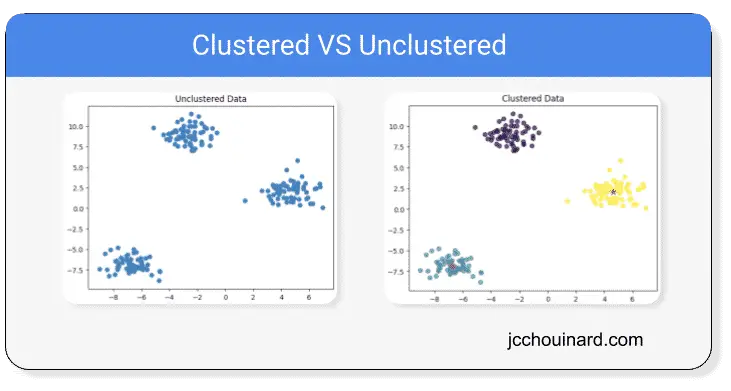
Example of Clustering Algorithms
Here are the 3 most popular clustering algorithms that we will cover in this article:
- KMeans
- Hierarchical Clustering
- DBSCAN
Below we show an overview of other Scikit-learn‘s clustering methods
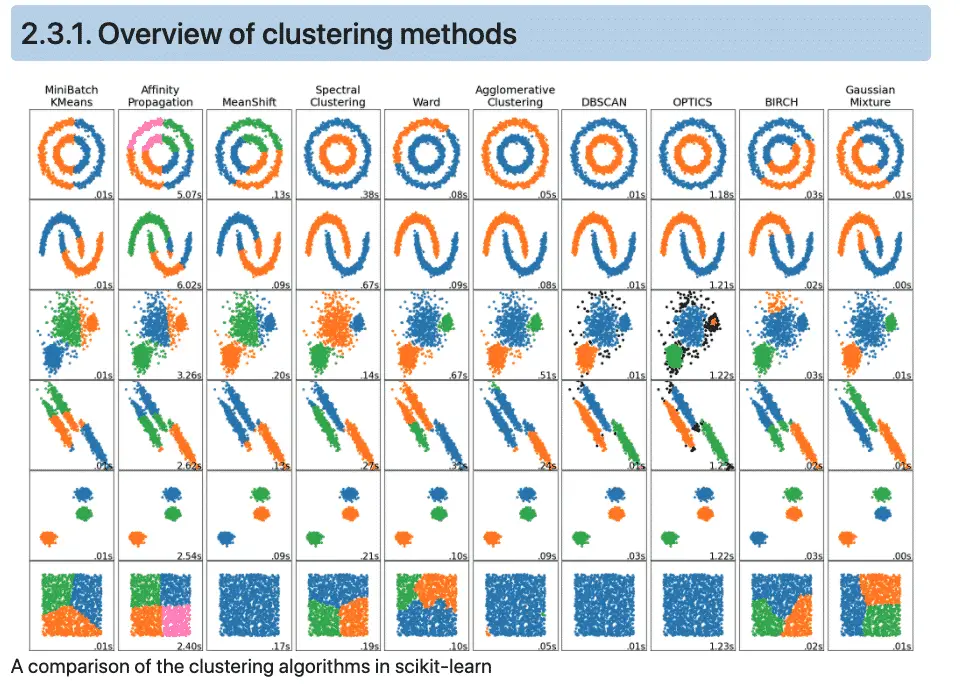
Examples of clustering problems
- Recommender systems
- Semantic clustering
- Customer segmentation
- Targetted marketing
How do clustering algorithms work?
Each clustering algorithm works differently than the other, but the logic of KMeans and Hierarchical clustering is similar. Clustering machine learning algorithm work by:
- Selecting cluster centers
- Computing distances between data points to cluster centers, or between each cluster centers.
- Redefining cluster center based on the resulting distances.
- Repeating the process until the optimal clusters are reached
This is an overly simplified view of clustering, but we will dive deeper into how each algorithm works specifically in the next sections.
How does KMeans Clustering Work?
Kmeans clustering algorithm works by starting with a fixed set of clusters and moving the cluster centres until the optimal clustering is met.
- Defining a number of clusters at the start
- Selecting random cluster centers
- Computing distances between each point to cluster center
- Finding new cluster centers using the mean of distances
- Repeating until convergence.
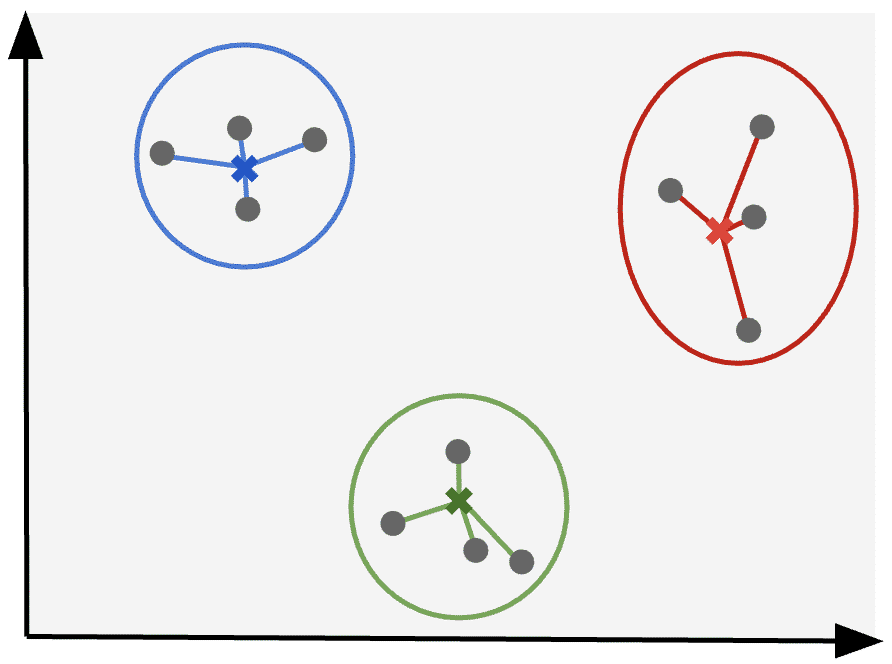
Some examples of KMeans clustering algorithms are:
KMeans
from Scikit-learn’ssklearn.cluster
kmeans
from SciPy’sscipy.cluster.vq
Kmeans Clustering in Python Example
from sklearn.datasets import make_blobs
from sklearn.cluster import KMeans
import matplotlib.pyplot as plt
plt.style.use('default')
# create a dataset of 200 samples
# and 5 clusters
features, labels = make_blobs(
n_samples=200,
centers=5
)
# Instantiate the model with 5 'K' clusters
# and 10 iterations with different
# centroid seed
model = KMeans(
n_clusters=5,
n_init=10,
random_state=42
)
# train the model
model.fit(features)
# make a prediction on the data
p_labels = model.predict(features)
# Plot the results
X = features[:,0]
y = features[:,1]
plt.scatter(X, y, c=p_labels, alpha=0.8)
cluster_centers = model.cluster_centers_
cs_x = cluster_centers[:,0]
cs_y = cluster_centers[:,1]
plt.scatter(cs_x, cs_y, marker='*', s=100, c='r')
plt.title('KMeans')
plt.show()
How does Hierarchical Clustering Work?
Hierarchical clustering algorithm works by starting with 1 cluster per data point and merging the clusters together until the optimal clustering is met.
- Having 1 cluster for each data point
- Defining new cluster centers using the mean of X and Y coordinates
- Combining clusters centers closest to each other
- Finding new cluster centers based on the mean
- Repeating until optimal number of clusters is met
The image below represents a dendrogram that can be used to visualize hierarchical clustering. Starting with 1 cluster per data point at the bottom and merging the closest clusters at each iteration, ending up with a single cluster for the entire dataset.
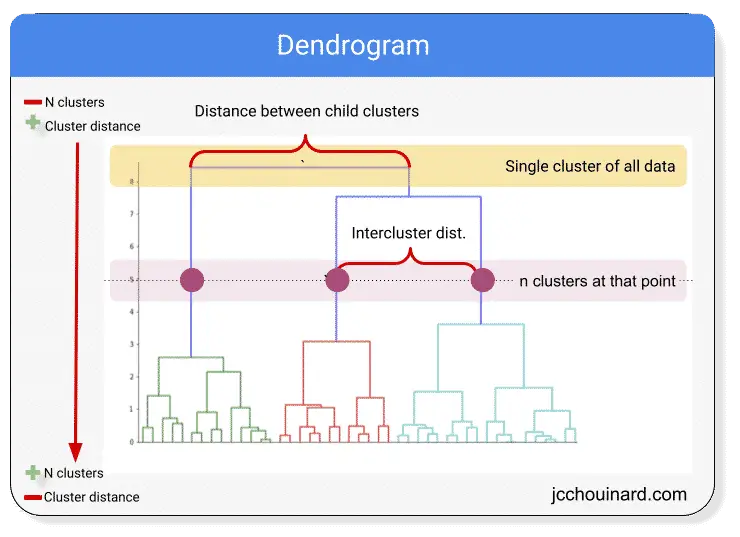
Some examples of hierarchical clustering algorithms are:
hirearchy
from SciPy’sscipy.cluster
Hierarchical Clustering in Python Example
import matplotlib.pyplot as plt
import numpy as np
from numpy.random import rand
import pandas as pd
import seaborn as sns
from scipy.cluster.vq import whiten
from scipy.cluster.hierarchy import fcluster, linkage
# Generate initial data
data = np.vstack((
(rand(30,2)+1),
(rand(30,2)+2.5),
(rand(30,2)+4)
))
# standardize (normalize) the features
data = whiten(data)
# Compute the distance matrix
matrix = linkage(
data,
method='ward',
metric='euclidean'
)
# Assign cluster labels
labels = fcluster(
matrix, 3,
criterion='maxclust'
)
# Create DataFrame
df = pd.DataFrame(data, columns=['x','y'])
df['labels'] = labels
# Plot Clusters
sns.scatterplot(
x='x',
y='y',
hue='labels',
data=df
)
plt.title('Hierachical Clustering with SciPy')
plt.show()
How does DBSCAN Clustering Work?
DBSCAN stands for Density-Based Spatial Clustering of Applications and Noise.
DBSCAN clustering algorithm works by assuming that the clusters are regions with high-density data points separated by regions of low-density.
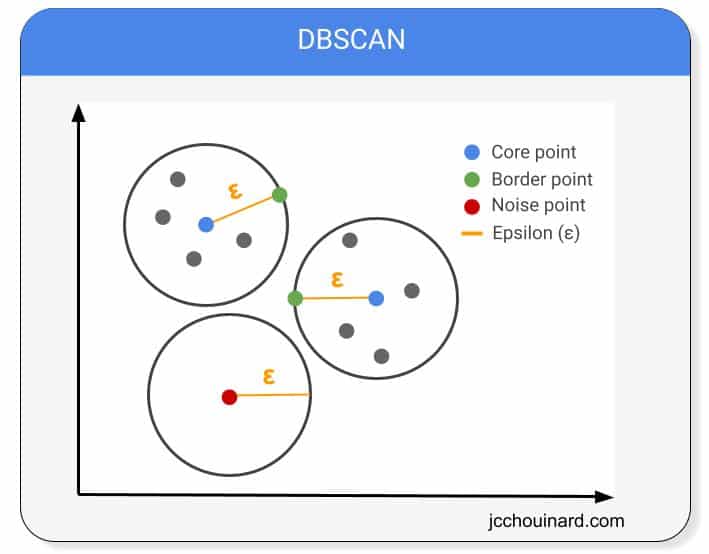
Some examples of DBSCAN clustering algorithms are:
DBSCAN
from Scikit-learnsklearn.cluster
- HDBSCAN
DBSCAN Clustering in Python Example
import numpy as np
import matplotlib.pyplot as plt
from sklearn.datasets import make_moons
from sklearn.cluster import DBSCAN
# Create a synthetic dataset with two moons
X, _ = make_moons(n_samples=200, noise=0.05, random_state=0)
# Apply DBSCAN
dbscan = DBSCAN(eps=0.3, min_samples=5)
cluster_labels = dbscan.fit_predict(X)
# Visualize the results
plt.scatter(X[:, 0], X[:, 1], c=cluster_labels, cmap='viridis')
plt.xlabel('Feature 1')
plt.ylabel('Feature 2')
plt.title('DBSCAN Clustering')
plt.show()
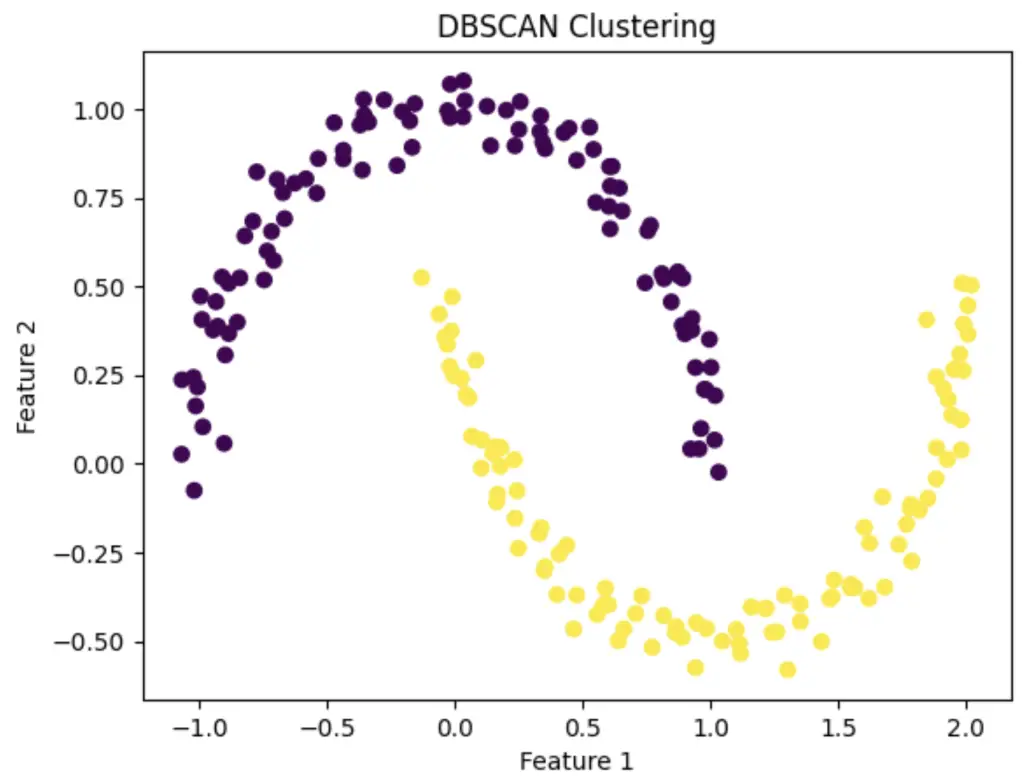
How does Gaussian Mixture Clustering Models Work?
Gaussian Mixture Models, or GMMs, are probabilistic models that look at Gaussian distributions, also known as normal distributions, to cluster data points together.
By looking at a certain number of Gaussian distributions, the models assume that each distribution is a separate cluster.
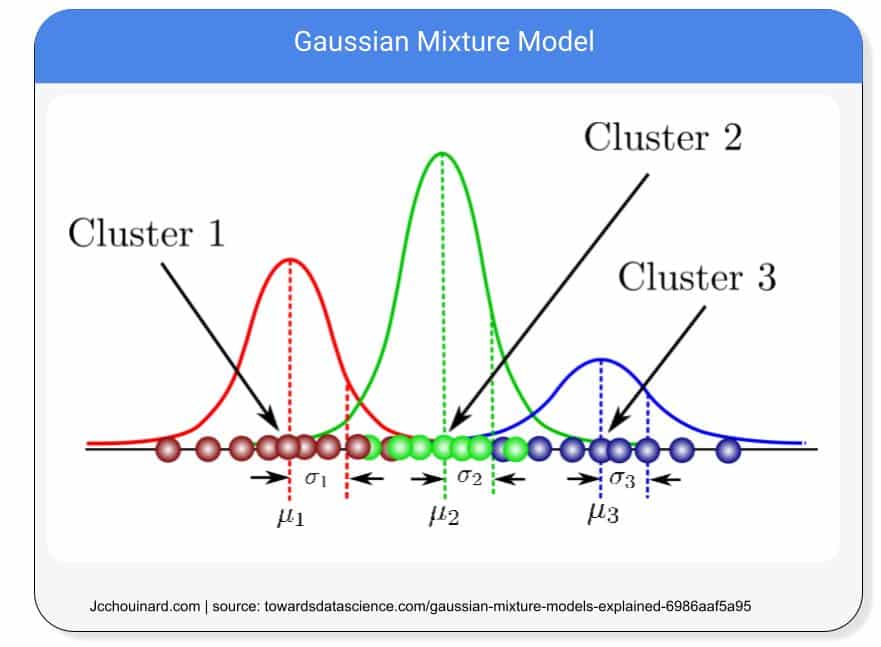
Some examples of Gaussian mixture clustering algorithms are:
GaussianMixture
from Scikit-learn’ssklearn.mixture
Gaussian Mixture Clustering in Python Example
import numpy as np
import matplotlib.pyplot as plt
from sklearn.datasets import make_blobs
from sklearn.mixture import GaussianMixture
# Generate a synthetic dataset with three clusters
X, _ = make_blobs(n_samples=300, centers=3, random_state=42)
# Fit a Gaussian Mixture Model to the data
gmm = GaussianMixture(n_components=3, random_state=42)
gmm.fit(X)
# Predict the cluster labels for each data point
labels = gmm.predict(X)
# Plot the data points with colors representing clusters
plt.scatter(X[:, 0], X[:, 1], c=labels, cmap='viridis')
plt.xlabel('Feature 1')
plt.ylabel('Feature 2')
plt.title('Gaussian Mixture Clustering')
plt.colorbar()
plt.show()
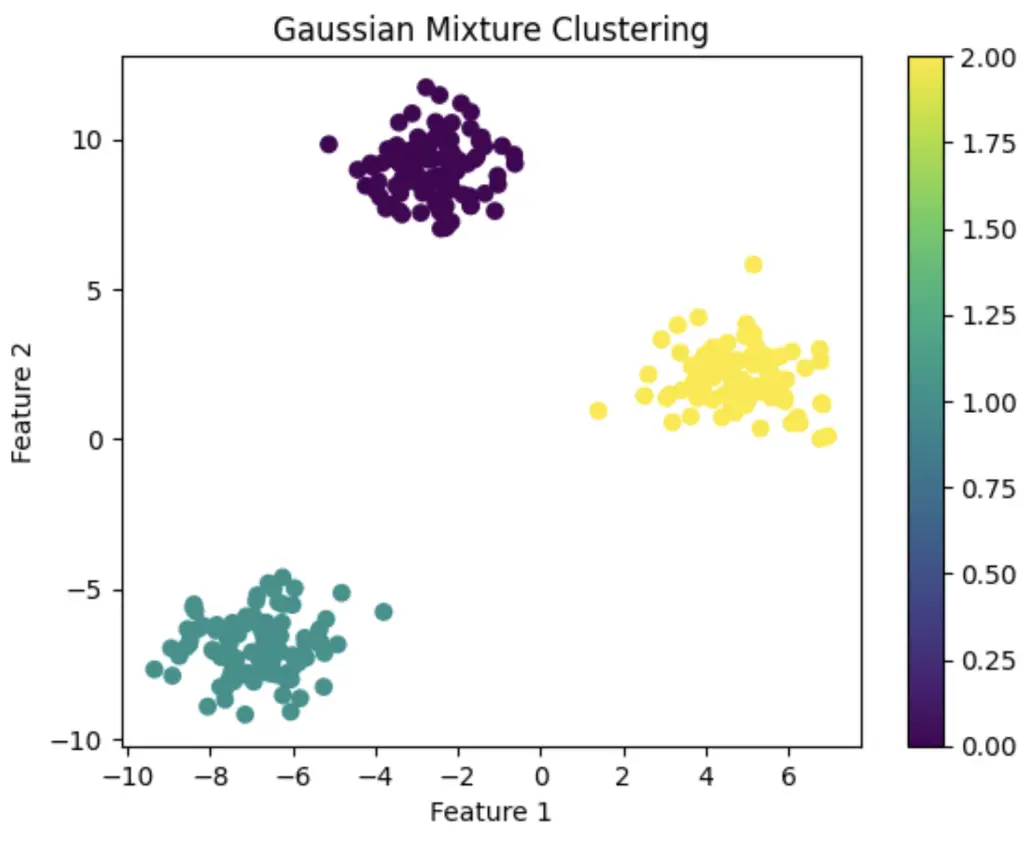
Interesting Work from the Community
How to Master the Popular DBSCAN Clustering Algorithm for Machine Learning by Abhishek Sharma
Build Better and Accurate Clusters with Gaussian Mixture Models
Python Script: Automatically Cluster Keywords In Bulk For Actionable Insights V2 by Lee Foot
Semantic Keyword Clustering Command-Line Interface Application by Lee Foot
Polyfuzz auto-mapping + auto-grouping tests by Charly Wargnier
How To Make Clustering in Machine Learning
To cluster data in Scikit-Learn using Python, you must process the data, train multiple classification algorithms and evaluate each model to find the classification algorithm that is the best predictor for your data
- Load data
You can load any labelled dataset that you want to predict on. For instance, you can use
fetch_openml('mnist_784')
on the Mnist dataset to practice. - Explore the dataset
Use python pandas functions such as
df.describe()
anddf.isnull().sum()
to find how your data need to be processed prior training - Preprocess data
Drop, fill or impute missing, or unwanted values from your dataset to make sure that you don’t introduce errors or bias into your data. Use pandas
get_dummies()
,drop()
, andfillna()
functions alongside some sklearn’s libraries such asSimpleImputer
orOneHotEncoder
to preprocess your data. - Split data into training and testing dataset
To be able to evaluate the accuracy of your models, split your data into training and testing sets using sklearn’s
train_test_split
. This will allow to train your data on the training set and predict and evaluate on the testing set. - Create a pipeline to train multiple clustering algorithms and hyper-parameters
Run multiple algorithms, and for each algorithm, try various hyper-parameters. This will allow to find the best performing model and the best parameters for that model. Use
GridSearchCV()
andPipeline
to help you with these tasks - Evaluate the machine learning model
Evaluate the model on its precision with methods such as the homogeneity_score() and completeness_score() and evaluate elements such as the confusion_matrix() in Scikit-learn
Classification and Machine Learning Definitions
Clustering in machine learning | Process of dividing objects into similar clusters |
Clustering examples | Recommender systems and semantic clustering |
Clustering algorithms | KMeans, Hierarchical Clustering and DBSCAN |
Clustering is used in | Clustering is a Supervised learning approach |
Libraries used for clustering | Scikit-learn is a popular library in clustering |
Conclusion
This concludes the introduction of clustering in machine learning. We have covered how clustering works and provided an overview of the most common clustering machine learning models.
The next step is to learn how to use Scikit-learn to train each clustering machine learning model on real data.
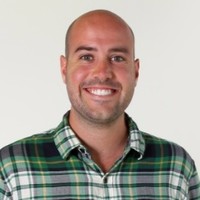
SEO Strategist at Tripadvisor, ex- Seek (Melbourne, Australia). Specialized in technical SEO. Writer in Python, Information Retrieval, SEO and machine learning. Guest author at SearchEngineJournal, SearchEngineLand and OnCrawl.