There are 11 main methods that can be used when working with a principal component analysis object in Python.
Here is a table listing all the methods that can be applied on the PCA object.
fit() | Fit the model with X. |
fit_transform() | Fit the model with X and apply the dimensionality reduction on X. |
get_covariance() | Compute data covariance with the generative model. |
get_feature_names_out() | Get output feature names for transformation. |
get_params() | Get parameters for this estimator. |
get_precision() | Compute data precision matrix with the generative model. |
inverse_transform() | Transform data back to its original space. |
score() | Return the average log-likelihood of all samples. |
score_samples() | Return the log-likelihood of each sample. |
set_params() | Set the parameters of this estimator. |
transform() | Apply dimensionality reduction to X. |
to learn more, read our tutorial on how to use PCA with Python.
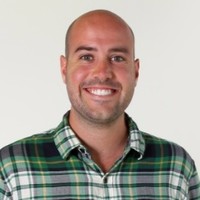
SEO Strategist at Tripadvisor, ex- Seek (Melbourne, Australia). Specialized in technical SEO. Writer in Python, Information Retrieval, SEO and machine learning. Guest author at SearchEngineJournal, SearchEngineLand and OnCrawl.